A New Kind of Risk?
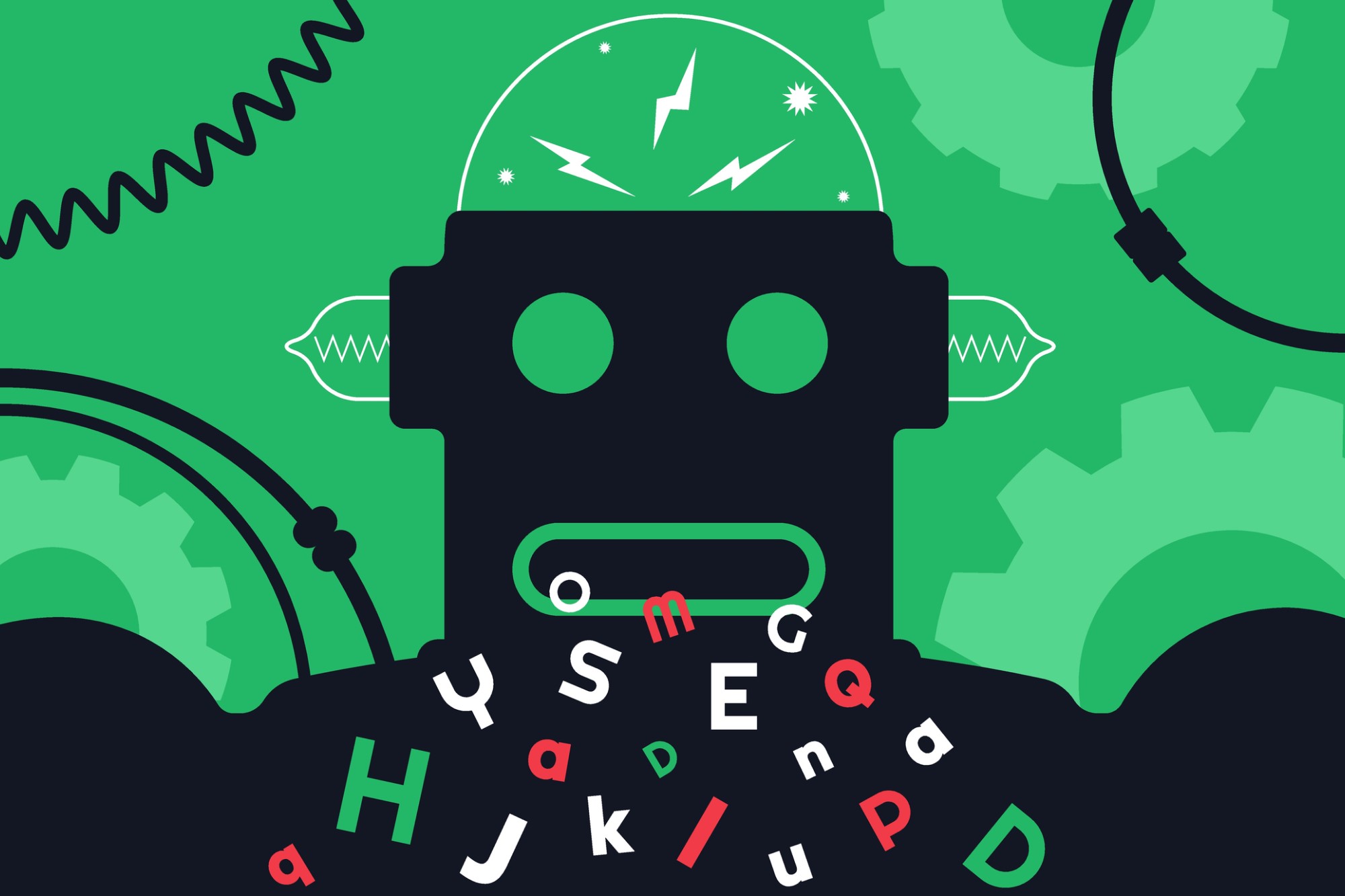
We usually expect to be held accountable for our actions – for both results we intend, and those we do not. We expect, for example, that a car company will ensure that a vehicle doesn’t have major flaws that could result in serious harm before they sell it to customers. To not consider the risks would be negligent, and this is why recalls often look bad for such companies.
But what about algorithms? Should we have a similar expectation that a corporation that develops an algorithm to detect cancer or to detect whether someone is passing off AI-generated content as their own should be sure that there are no significant flaws in their product before they sell it? What if there is no way they could reasonably do so? Given that algorithms can generate erroneous results resulting in serious harms, what is a reasonable standard when it comes to product testing?
In one of the chapters of my forthcoming book on the ethics of AI, I consider a hypothetical issue involving ChatGPT and a professor who might use an algorithm to accuse a student of passing off ChatGPT-written work as their own. There are a great many ethical issues involved when we don’t understand the algorithm and how it might generate false positive results. This has already become a serious issue as students are now being falsely accused of handing in AI-generated work because an algorithm flagged it. A Bloomberg Businessweek study on the services GPTZero and Copyleaks found a 1-2% false positive rate. While that may not sound like a lot, it can mean that millions of students will be falsely accused of cheating with almost no way of defending themselves or receiving an explanation as to what they did wrong.
According to Bloomberg, these interactions are already ruining academic relationships between teachers and students. Some students have now taken to recording themselves writing their entire papers just to be able to disprove the algorithm. Others now obsess about not writing “too robotic” lest they be accused themselves, a problem that is especially prominent for ESL and neuro-divergent students. Should we hold the AI developer whose faulty product generates these kinds of results negligent?
Philosophers of science generally agree that researchers have an obligation to assess inductive risk concerns when accepting a conclusion. In other words, they need to consider what the moral consequences of potentially getting it wrong might be and then consider whether a higher or lower standard of evidence might be appropriate. If, for example, we were testing a chemical to determine how hazardous it is, but the test was only accurate 80% of the time, we would likely demand more evidence. Given the potential harm that can result and the opaqueness of algorithms, AI developers should be similarly conscientious.
If an algorithm operates according to black box principles, the developer may have a good understanding of how to create an algorithm – they will understand that the model can take in various inputs and translate those into outputs – but they will not be able to retrace the steps the model used to arrive at its conclusion. In other words, we have no idea what evidence an algorithm like GPTZero is relying on when it concludes that a piece of text is generated by AI. If the AI developer doesn’t know how the algorithm is using input data as evidence, they cannot evaluate the inductive risk concerns about how sufficient that evidence is.
Still, there are ways, despite the opacity, that an AI developer might attempt to address their inductive risk responsibilities. Koray Karaca argues that developers can build in inductive risk by using cost sensitive machine learning by assigning different costs to different kinds of errors. In the case of AI detectors, the company Turnitin claims to intentionally “oversample” underrepresented students (especially ESL students). By oversampling in this way, the evidentiary standard by which different forms of writing are judged is fine tuned.
Still, there is little accounting for what correlations a model might rely on, making it difficult to explain to students who do get falsely accused why they are being accused in the first place. AI developers have struggled to assess the reliability of their models or evaluate the risks when those correlations are used in error. This issue becomes especially concerning when it comes to things like credit reports. If you don’t know how or why a model compiles a credit report, how can you manage those risks of error? How much must a developer understand about how their algorithm functions before it is put to use? If a developer is aware of the risks of error but also knows that their algorithm is limited in terms of mitigating those risks, at what point do we consider that negligent behavior? If negligence is essentially something we police as a community, we will need to come together quickly to decide what the promise of AI can and can’t excuse.