Should AI Reflect Us as We Are or as We Wish to Be?
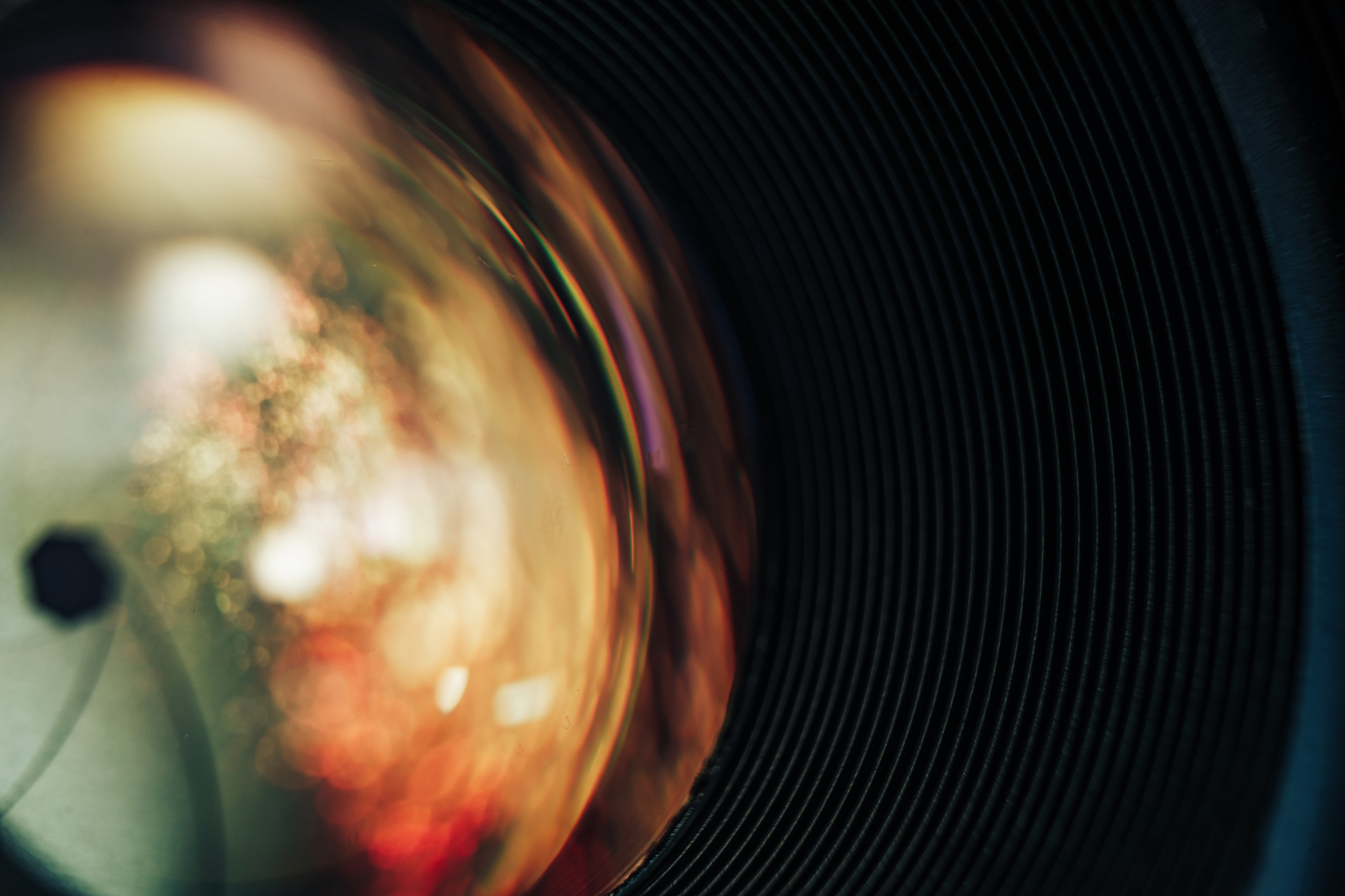
Our understanding of AI has come a very long way in a short amount of time. But one issue we still have yet to crack is the prevalence of bias. And this seems especially troubling since AI does everything from determining if you should go to jail, to whether you get a job, to whether you should receive healthcare, and more. Efforts have been made to make algorithms less biased – like including greater diversity in training data – but issues persist. Recently, Google had to suspend their Gemini AI platform because of the images it was generating. Users reported that when they asked for pictures of Nazi soldiers in 1943, they would get images of multi-ethnic people in Nazi uniforms. Another user requested a picture of a medieval British king and received equally counterfactual content. Clearly our desire to combat social bias conflicts with our desire for accuracy. How should problems like this be addressed?
There are good reasons for wanting to prevent AI from producing content that reflects socially harmful bias. We don’t want it to simply reinforce past prejudice. We don’t want only images of men as doctors and lawyers and images of women as secretaries and nurses. If biases like these were systematic across AI, it could perpetuate social stereotypes. Presumably, we might instead desire that if we asked for images of a CEO at work, that a significant portion of the images would be women (regardless of past statistics).
A similar concern occurs when we consider generative AI’s handling of race. In order for algorithms to generate an image, it requires large amounts of training data to pull from. However, if there are biases in the training data, this can lead to biased results as well. If the training data contains mostly images of people with white skin and few images of people with black or brown skin, the algorithm will be less likely to generate images of black or brown skinned people in images and may struggle to reproduce different ethnic facial features. Research on facial recognition algorithms, for example, has demonstrated how difficult it can be to discern different skin tones without a diverse training dataset.
Correcting for these problems requires that developers be mindful of the kinds of assumptions they make when designing an algorithm and curating training data. As Timnit Gebru – who famously left Google over a dispute about ethical AI – has pointed out, “Ethical AI is not an abstract concept but is one that is in dire need of a holistic approach. It starts from who is at the table, who is creating the technology, and who is framing the goals and values of AI.” Without a serious commitment to inclusion, it will be impossible to catch bias before it gets reproduced again and again. It’s a system of garbage in, garbage out.
While biased AI can have real life significant impacts on people – such as the woman who lost her refugee status after a facial recognition algorithm failed to properly identify her, or the use of predictive policing and recidivism algorithms that tend to target Black people – there’s also the risk that in attempting to cleanse real-life biases from AI we distort reality. The curation of training data is a delicate balance. Attempts to purge the presence of bias from AI can go too far. The results may increasingly reflect the world as we ideally imagine it rather than as it actually is.
The Google Gemini controversy demonstrates this clearly: In attempting to create an algorithm featuring diverse people, it generates results that are not always true to life. If we return to the example of women CEOs, the problem is clearer. If someone performs a google image search of CEOs, it might mostly generate images of men and we might object that this is biased. Surely if a young person were to look up images of CEOs, we would want them to find examples other than men. Yet, in reality, women account for about ten percent of CEOs of fortune 500 companies. But, if the impression the public gets is the opposite, that women make up a far more significant number of CEOs than they actually do, they may not realize the real-life bias that exists. By curating an ideal AI version of our world, we cover up problems and become less aware of real-life bias and are less prepared to resolve those problems.
Consider an example like predictive policing where algorithms are often trained using crime data collected through biased policing. While we can attempt to correct the data, we should also be reminded of our responsibility to correct those practices in the first place. The reason an algorithm may not produce an image of a female CEO or that an algorithm predicts crime in poor neighborhoods is not the algorithm’s fault, it simply reflects what it sees. Correcting for bias in data may eventually go a long way towards correcting bias in society, but it can also create problems by distorting our understanding of society. There is moral risk in deciding the degree to which we want AI to reflect our own human ugliness back at us and the degree to which we want it to reflect something better.