Specious Content and the Need for Experts
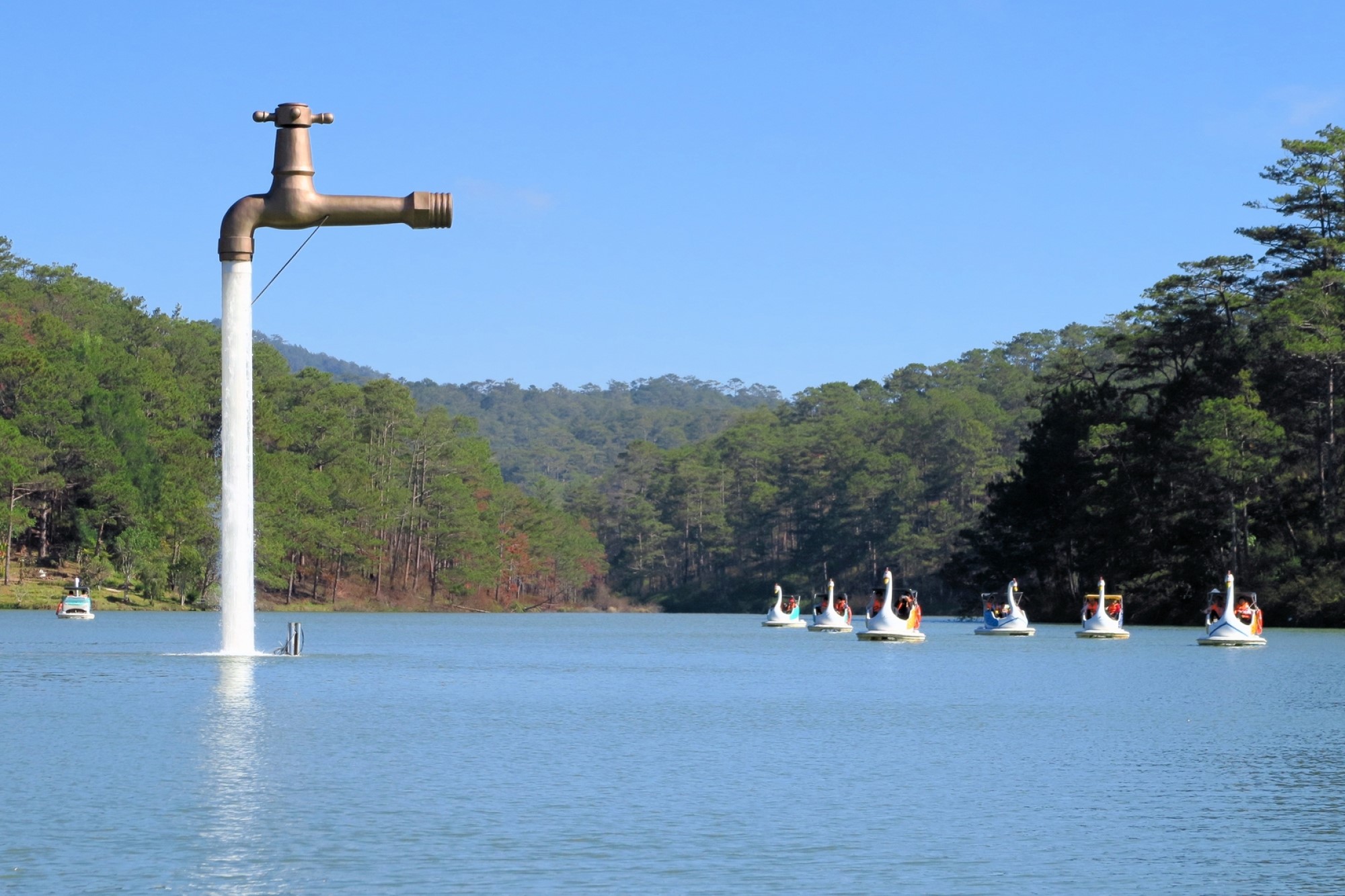
A recent tweet shows what looks to be a photo of a woman wearing a kimono. It looks authentic enough, although not knowing much about kimonos myself I couldn’t tell you much about it. After learning that the image is AI-generated, my opinion hasn’t really changed: it looks fine to me, and if I ever needed to use a photo of someone wearing a kimono, I may very well choose something that looked the same.
However, reading further we see that the image is full of flaws. According to the author of the tweet who identifies themselves as a kimono consultant, the fabric doesn’t hang correctly, and there are pieces seemingly jutting out of nowhere. Folds are in the wrong place, the adornments are wrong, and nothing really matches. Perhaps most egregiously, it is styled in a way that is reserved only for the deceased, which would make the person someone who was committing a serious faux pas, or a zombie.
While mistakes like these would fly under the radar of the vast majority of those viewing it, it’s indicative of the ability of AI-powered generative image and text programs to produce content that appears authoritative but is riddled with errors.
Let’s give this kind of content a name: specious content. It’s the kind of content – be it in the form of text, images, or video – that appears to be plausible or realistic on its surface, but is false or misleading in a way that can only be identified with some effort and relevant knowledge. While there was specious content before AI programs became ubiquitous, the ability of such programs to produce content on a massive scale for free significantly increases the likelihood of misleading users and causing harm.
Given the importance of identifying AI-generated text and images, what should our approach be when dealing with content that we suspect is specious? The most common advice seems to be that we should rely on our own powers of observation. However, this approach may very well do more harm than good.
A quick Googling of how to avoid being fooled by AI-generated images will turn up much of the same advice: look closely and see if anything looks weird. Media outlets have been quick to point out that AI image-generating tools often mess up hands and fingers, that sometimes glasses don’t quite fit right on someone’s face, or that body parts or clothes overlap in places where they shouldn’t. A recent New York Times article goes even further and suggests that people look for mismatched fashion accessories, eyes that are too symmetrically spaced, glasses with mismatching end pieces, indents in ears, weird stuff in someone’s hair, and a blurred background.
The problem with all these suggestions is that they’re either so obvious as to not be worth mentioning, or so subtle that they would escape noticing even under scrutiny.
If an image portrays someone with three arms you are probably confident enough already that the image isn’t real. But people blur their backgrounds on purpose all the time, sometimes they have weird stuff in their hair, and whether a face is “too symmetrical” is a judgment beyond the ability of most people.
A study recently discussed in Scientific American underscores how scrutinizing a picture for signs of imperfections is a strategy that’s doomed to fail. It found that while participants performed no better than chance at identifying AI-generated images without any instruction, their detection rate increased by a mere 10% after reading advice on how to look closely for imperfections. With AI technology getting better every day, it seems likely that even these meager improvements won’t last long.
We’re not only bad at analyzing specious content, but going through checklists of subtle indicators is just going to make things worse. The problem is that it’s easy to interpret the lack of noticeable mistakes as a mark of authenticity: if we are unable to locate any signs that an image is fake, then we may be more likely to think that it’s genuine, even though the problems may be too subtle for us to notice. Or we might simply not be knowledgeable or patient enough to find them. In the case of the kimono picture, for example, what might be glaringly obvious to someone who was familiar with kimonos goes straight over my head.
But these problems also guide us to better ways of dealing with specious content. Instead of relying on our own limited capacity to notice mistakes in AI-generated images, we should outsource these tasks.
One new approach to detecting these images comes from AI itself: as tools to produce images have improved, so have tools that have been designed to detect those images (although it seems as though the former is winning, for now).
The other place to look for help is from experts. Philosophers debate about what, exactly, makes an expert, but in general, they typically possess a lot of knowledge and understanding of a subject, make reliable judgments about matters within their domain of expertise, are often considered authoritative, and can explain concepts to others. While identifying experts is not always straightforward, what will perhaps become a salient marker of expertise in the current age of AI will be one’s ability to distinguish specious content from that which is trustworthy.
While we certainly can’t get expert advice for every piece of AI-generated content we might come across, increasing amounts of authoritative-looking nonsense should cause us to recognize our own limitations and attempt to look to those who possess expertise in a relevant area. While even experts are sometimes prone to being fooled by AI-generated content, the track record of non-experts should lead us to stop looking closely for weird hands and overly-symmetrical features and start looking for outside help.