High Theory and Ethical AI
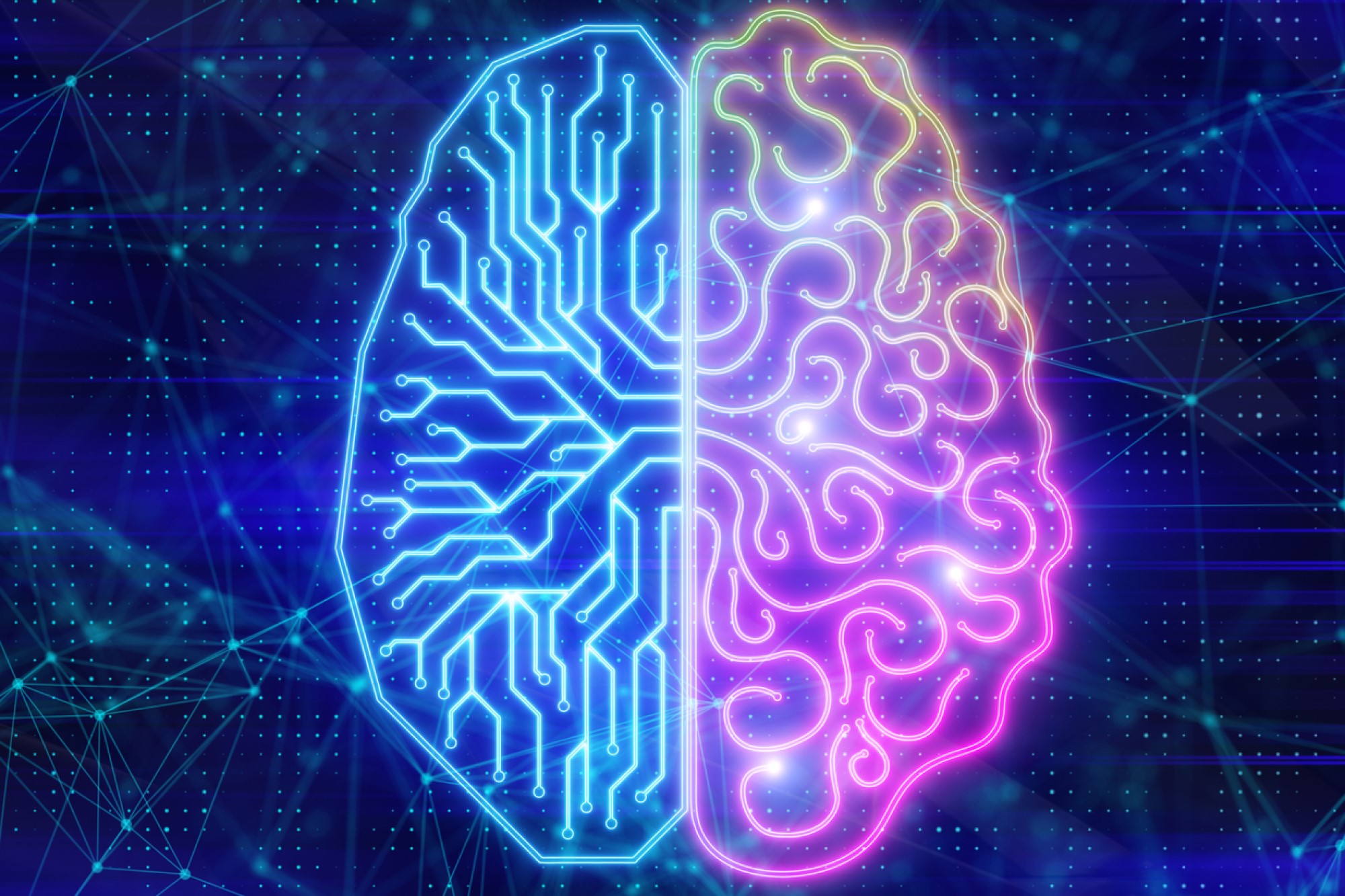
There’s been a push to create ethical AI through the development of moral principles embedded into AI engineering. But debate has recently broken out as to what extent this crusade is warranted. Reports estimate that there are at least 70 sets of ethical AI principles proposed by governments, companies, and ethics organizations. For example, the EU adopted its Ethical Guidelines for Trustworthy AI which prescribes adherence to four basic principles: respect for human autonomy, prevention of harm, as well as a commitment to fairness and explicability.
But critics charge that these precepts are so broad and abstract as to be nearly useless. Without clear ways to translate principle into practice, they are nothing more than hollow virtue signaling. Who’s right?
Because of the novel ethical issues that AI creates, there aren’t pre-existing ethical norms to govern all use cases. To help develop ethics governance, many bodies have borrowed a “high theory” approach from bioethics – solving ethical problems involves the application of abstract (or “high”) ethical principles to specific problems. For example, utilitarianism and deontology are usually considered high level theories and a high theory approach to bioethics would involve determining how to apply these principles in specific cases. In contrast, a low theory approach is built from the ground up by looking at individual cases first instead of principles.
Complaints about the overreliance on principles in bioethics are well known. Steven Toulmin’s “The Tyranny of Principles” notes how people can often agree on actions, but still disagree about the principle. Brent Mittelstadt has argued against high theory approaches in AI because of the logistical issues that separates tech ethics from bioethics. He notes, for example, that unlike medicine which has always has the common aim of promoting health of a patient, AI development has no common aim.
AI development is not a formal profession that entails certain fiduciary responsibilities and obligations. There is no notion of what a “good” AI developer is relative to a “good” doctor.
As Mittelstadt emphasizes, “the absence of a fiduciary relationships in AI means that users cannot trust that developers will act in their best interests when implementing ethical principles in practice.” He also argues that unlike medicine where the effects of clinical decision-making are often immediate and observable, the impact of decisions in AI development may never be apparent to developers. AI systems are often opaque in the sense that no one person has a full understanding of the system’s design or function. The difficulty of tracing decisions, impacts, and ethical responsibilities for various decisions becomes incredibly confusing. For similar reasons, the broad spectrum of actors involved in AI development, all coming from different technical and professional backgrounds, means that there is no common culture to ensure that abstract principles are collectively understood. Making sure that AI is “fair,” for example, would not be specific enough to be action-guiding for all contributors regarding development and end-use.
Consider the recent case of the AI rapper who given a record deal only to have the deal dropped after a backlash over racial stereotypes, or the case of the AI who recently won an art contest over real artists and all the developers involved in making those projects possible.
Is it likely they share a common understanding of a concept like prevention of harm, or a similar way of applying it? Might special principles apply to things like the creation of art?
Mittelstadt points out that high level principles are uniquely applicable in medicine because there are proven methods in the field to translate principles into practice. All those professional societies, ethics review boards, licensing schemes, and codes of conduct help to do this work by comparing cases and identifying negligent behavior. Even then, high level principles rarely explicitly factor into clinical decision-making. By comparison, the AI field has no similar shared institutions to allow for the translation of high-level principles into mid-level codes of conduct, and it would have to factor in elements of the technology, application, context of use, and local norms. This is why even as new AI ethics advisory boards are created, problems persist. While these organizations can prove useful, they also face immense challenges owing to the disconnect between developers and end users.
Despite these criticisms, there are those who argue that high-level ethical principles are crucial for developing ethical AI. Elizabeth Seger has argued that building the kinds of practices that Mittelstadt indicates require a kind of “start-point” that moral principles can provide. Those principles provide a road map and suggest particular avenues for further research.
They represent a first step towards developing the necessary practices and infrastructure, and cultivate a professional culture by establishing behavioral norms within the community.
High-level AI principles, Seger argues, provide a common vocabulary AI developers can use to discuss design challenges and weigh risks and harms. While AI developers already follow principles of optimization and efficiency, a cultural shift around new principles can augment the already existing professional culture. The resulting rules and regulations will have greater efficacy if they appeal to cultural norms and values held by the communities they are applied to. And if the professional culture is able to internalize these norms, then someone working in it will be more likely to respond to the letter and spirit of the policies in place.
It may also be the case that different kinds of ethical problems associated with AI will require different understandings of principles and different application of them during the various stages of development. As Abhishek Gupta of the Montreal AI Ethics Institute has noted, the sheer number of sets of principles and guidelines that attempt to break down or categorize subdomains of moral issues presents an immense challenge. He suggests categorizing principles according to the specific areas – privacy and security, reliability and safety, fairness and inclusiveness, and transparency and accountability – and working on developing concrete applications of those principles within each area.
With many claiming that adopting sets of ethics principles in AI is just “ethics washing,” and with AI development being so broad, perhaps the key to regulating AI is not to focus on what principles should be adopted, but to focus on how the AI development field is organized. It seems like whether we start with high theory or not, getting different people from different backgrounds to speak a common ethics language is he first step and one that may require changing the profession of AI development itself.