Correcting Bias in A.I.: Lessons from Philosophy of Science
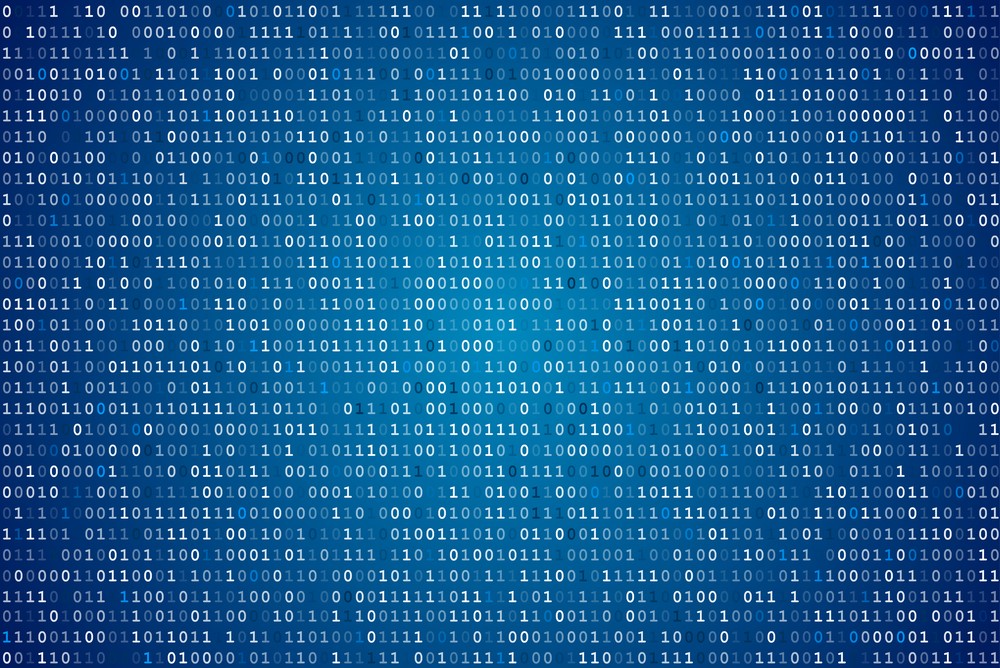
One of the major issues surrounding artificial intelligence is how to deal with bias. In October, for example, a protest was held by Uber drivers, decrying the algorithm the company uses to verify its drivers as racist. Many Black drivers were unable to verify themselves because the software fails to recognize them. Because of this, many drivers cannot get verified and are unable to work. In 2018, a study showed that a Microsoft algorithm failed to identify 1 in 5 darker-skinned females, and 1 in 17 darker-skinned males.
Instances like these prompt much strategizing about how we might stamp out bias once and for all. But can you completely eliminate bias? Is the solution to the problem a technical one? Why does bias occur in machine learning, and are there any lessons that we can pull from outside the science of AI to help us consider how to address such problems?
First, it is important to address a certain conception of science. Historically, scientists – mostly influenced by Francis Bacon – espoused the notion that science was purely about investigation into the nature of the world for its own sake in an effort to discover what the world is like from an Archimedean perspective, independent of human concerns. This is also sometimes called the “view from nowhere.” However, many philosophers who would defend the objectivity of science now accept that science is pursued according to our interests. As philosopher of science Philip Kitcher has observed, scientists don’t investigate any and all forms of true claims (many would be pointless), but rather they seek significant truth, where what counts as significant is often a function of the interests of epistemic communities of scientists.
Next, because scientific modeling is influenced by what we take to be significant, it is often influenced by assumptions we take to be significant, whether there is good evidence for them or not. As Cathy O’Neil notes in her book Weapons of Math Destruction, “a model…is nothing more than an abstract representation of some process…Whether it’s running in a computer program or in our head, the model takes what we know and uses it to predict responses to various situations.” Modeling requires that we understand the evidential relationships between inputs and predicted outputs. According to philosopher Helen Longino, evidential reasoning is driven by background assumptions because “states of affairs…do not carry labels indicating that for which they are or for which they can be taken as evidence.”
As Longino points out in her book, often these background assumptions cannot always be completely empirically confirmed, and so our values often drive what background assumptions we adopt. For example, clinical depression involves a myriad of symptoms but no single unifying biological cause has been identified. So, what justifies our grouping all of these symptoms into a single illness? According to Kristen Intemann, what allows us to infer the concept “clinical depression” from a group of symptoms are assumptions we have that these symptoms impair functions we consider essential to human flourishing, and it is only through such assumptions that we are justified in grouping symptoms with a condition like depression.
The point philosophers like Intemann and Longino are making is that such background assumptions are necessary for making predictions based off of evidence, and also that these background assumptions can be value-laden. Algorithms and models developed in AI also involve such background assumptions. One of the bigger ethical issues involving bias in AI can be found in criminal justice applications.
Recidivism models are used to help judges assess the danger posed by each convict. But people do not carry labels saying they are recidivists, so what would you take as evidence that would lead you to conclude someone might become a repeat offender? One assumption might be that if a person has had prior involvement with the police, they are more likely to be a recidivist. But if you are Black or brown in America where stop-and-frisk exists, you are already disproportionately more likely to have had prior involvement with the police, even if you have done nothing wrong. So, because of this background assumption, a recidivist model would be more likely to predict that a Black person is going to be a recidivist than a white person who is less likely to have had prior run-ins with the police.
But whether the background assumption that prior contact with the police is a good predictor of recidivism is questionable, and in the meantime this assumption creates biases in the application of the model. To further add to the problem, as O’Neil notes in her analysis of the issue, recidivism models used in sentencing involve “the unquestioned assumption…that locking away ‘high-risk’ prisoners for more time makes society safer,” adding “many poisonous assumptions are camouflaged by math and go largely untested and unquestioned.”
Many who have examined the issue of bias in AI often suggest that the solutions to such biases are technical in nature. For example, if an algorithm creates a bias based on biased data, the solution is to use more data to eliminate such bias. In other cases, attempts to technically define “fairness” are used where a researcher may require models that have equal predictive value across groups or require an equal number of false and negative positives across groups. Many corporations have also built AI frameworks and toolkits that are designed to recognize and eliminate bias. O’Neil notes how many responses to biases created by crime prediction models simply focus on gathering more data.
On the other hand, some argue that focusing on technical solutions to these problems misses the issue of how assumptions are formulated and used in modeling. It’s also not clear how well technical solutions may work in the face of new forms of bias that are discovered over time. Timnit Gebru argues that the scientific culture itself needs to change to reflect the fact that science is not pursued as a “view from nowhere.” Recognizing how seemingly innocuous assumptions can generate ethical problems will necessitate greater inclusion of people from marginalized groups. This echoes the work of philosophers of science like Longino who assert that not only is scientific objectivity a matter of degree, but science can only be more objective by having a well-organized scientific community centered around the notion of “transformative criticism,” which requires a great diversity of input. Only through such diversity of criticism are we likely to reveal assumptions that are so widely shared and accepted that they become invisible to us. Certainly, focusing too heavily on technical solutions runs the risk of only exacerbating the current problem.